How to Harness AI Data Governance for Data Integrity
It’s no secret that artificial intelligence (AI) is revolutionizing the way companies operate with its ability to sift through mountains of data and make accurate predictions at record speed. But with great power comes great responsibility.
As AI systems are more regularly incorporated into business, it’s critical that data sources are both accurate and secure to prevent error. That's why AI data governance — a strategic blueprint for managing data in an AI system — is the new frontier for modern companies.
What is AI data governance?
AI data governance is a systematic framework that governs the deployment, optimization, and management of AI data and technology within an organization. This process involves setting up steps for how to collect, store, and analyze data in AI systems while adhering to legal and ethical compliance standards.
The ultimate goals of AI data governance? To maintain the integrity of a company’s data, prevent security and privacy issues like data leaks, and maximize the benefits of automation.
Why a good AI data governance strategy matters
Without governance frameworks to define roles and responsibilities, monitor data activity, and create lasting accountability among teams, your organization’s data becomes vulnerable. And without an AI data governance strategy to help you adapt to a changing business environment, you may not be able to take full advantage of your data or capitalize on budding business opportunities.
In short, integrating a robust layer of AI data governance into your programs, processes, and company culture can help your organization build sustained success.
Privacy and security
AI data governance has the power to radically transform your organization’s data protection and privacy. Ensuring the integrity of your data not only increases your organization’s reliability and trustworthiness, but it also supports your adherence to the legal and ethical standards of governance frameworks like data regulations, which serve to protect personal identification and financial information. Robust data management enables your AI tools to house this information on a need-to-know basis with access limits and encrypt it to secure sensitive information.
Quality and integrity
For AI to perform at its best—offering sharp insights and making reliable choices—it needs an input of completely accurate data that is free of mistakes or biases. When the quality of your data isn't up to snuff, you end up with AI models that give faulty results. But with this proper input, these technologies can enhance your data quality while ensuring that your systems operate ethically and transparently. What’s more, AI can automate data classification and track lineage to ensure complete accuracy in seconds — allowing your organization to identify data patterns and anomalies faster.
Operational efficiency
AI can automate many of the manual tasks associated with data management, including classification, compliance checks, and policy enforcement, amongst others. By cutting down on these tasks, organizations can divert their time and resources spent on data analysis and strategic planning.
Furthermore, AI data governance frameworks can actually grow with your organization and ensure that data management practices remain efficient, leading to more stakeholder trust, smoother operations, and better accountability amongst team members.
Use cases for AI data governance in your business
Enterprise
It’s clear that using AI data in your business model can help companies make business decisions with informed insights to improve risk management — and ultimately result in greater profits. As such, this is a selling point, and your enterprise customers can have confidence that they’re getting the greatest possible value with full data optimization and project personalization.
Small and mid-sized businesses (SMBs)
AI data governance doesn’t just help support the big players, either. For small and mid-sized businesses (SMBs), it represents an important pathway to growth by helping carve out an advantage over competitors.
AI data governance can help SMBs categorize data and reach compliance using less resources, improving management and operation processes as they scale. This can help set these companies apart from others in the space and attract more clients.
Preparing for AI data governance
Successfully implementing AI data governance in your company doesn’t happen by accident. There are a few preparation items that help you assess your current data needs and make AI systems work for you.
Identify your needs
Before bringing AI into the mix, you first need to pinpoint what your data governance needs are. Start by asking what your data does, why you’re collecting it, and where it comes from. These pieces of information will give you a better sense of what your organization’s “data weak points” are, as well as how it can use AI systems to reach its goals.
Establish clear policies
Setting up clear rules, policies, and processes for managing data is crucial for data stewardship. This creates transparency around who can access data and how it can be used to help meet legal and ethical standards. We all know AI is getting smarter, but it must also be ethical and fair in its everyday use to comply with mandated standards.
Gather tools and resources
Finally, you can begin acquiring the right set of tools to implement AI data governance. These include data management platforms that offer capabilities for data cataloging, lineage tracking, quality checking, and metadata management to help organize your data landscape. Additionally, automated compliance and policy enforcement tools can align your data with regulatory requirements and standards in seconds. You should also pool your resources to oversee the implementation and management of AI in your company and add on data stewards and governance officers.
API Management and AI Data Governance
Kong serves as a compelling use case for the application of AI Data Governance by providing the necessary infrastructure to enforce data governance policies in environments where AI and machine learning (ML) models are deployed. In this context, AI Data Governance entails the strategies and practices that ensure data used by AI systems is handled securely, ethically, and in compliance with regulatory standards. Here’s how Kong facilitates AI Data Governance:
Secure Data Handling and Compliance
Kong’s API Gateway acts as the frontline defense for data entering and leaving AI systems, ensuring secure data handling practices are enforced. It employs robust security measures such as SSL/TLS encryption, authentication, and authorization to protect sensitive data, including the inputs and outputs of AI/ML models. By doing so, Kong helps organizations meet the stringent data protection requirements of regulations like GDPR and CCPA, which are critical components of AI Data Governance.
Fine-Grained Access Control
Kong allows for fine-grained access control over who can access AI services and data. By utilizing Kong’s capabilities to create specific policies for different user groups and services, organizations can ensure that only authorized personnel or systems have access to sensitive AI data. This level of control is essential for maintaining data privacy and ensuring that AI systems are not misused.
Data Flow Management
Kong facilitates the management of data flows between services, including those powered by AI. Through routing, transforming, and aggregating data, Kong ensures that data governance policies are applied consistently across the entire data lifecycle. This includes controlling how data is shared between microservices or external systems, essential for compliance and ethical AI use.
Auditability and Transparency
Through comprehensive logging and monitoring features, Kong provides audit trails for all API transactions, including those involving AI systems. This transparency is crucial for AI Data Governance, as it allows organizations to demonstrate compliance with data governance policies and regulations. It also enables the tracing of decisions made by AI systems back to the underlying data, supporting accountability and explainability in AI operations.
Plugin Ecosystem for Custom Governance Solutions
Kong’s extensible API platform supports the integration of custom plugins that can further enhance AI Data Governance. For example, organizations can develop plugins that anonymize sensitive data before it’s processed by AI models or that implement additional data validation checks. This adaptability allows organizations to tailor their AI governance strategies to their specific needs.
In essence, Kong empowers organizations to implement robust AI Data Governance frameworks by providing secure, compliant, and manageable infrastructure for AI and ML operations. By leveraging Kong’s capabilities, organizations can ensure their use of AI remains responsible, ethical, and aligned with both internal policies and external regulatory requirements.

How to implement AI data governance
Ready to jump in? While this process will differ between companies, we’ve created a step-by-step guide to help make sure you’re on track to a successful AI data governance strategy.
Step 1: Assess current data management practices
As we mentioned, you need to perform a data audit before diving headfirst into AI. This includes taking stock of where you source your data, how you manage it, and how it’s deployed across your business.
First, go through your data collection processes to check for consistency and reliability — and to find out what kind of data you’ve even got coming in. Then, take a closer look at how that data is stored and managed, paying close attention to who can access it, to get better insights into how AI can streamline your workflows.
Step 2: Define data quality and integrity goals
Now that you know where your data stands, you can set clear goals for quality and integrity. By coming up with guidelines and metrics for data optimization, you can create a more accurate, complete, reliable, and timely culture around your data. These targets will also help you decide which areas to implement AI and keep track of your progress so can you tweak the technology to work for you.
Step 3: Develop a compliance framework
We don’t recommend implementing AI before creating a set of rules to manage AI data. This compliance framework will ensure that your workforce establishes the roles and responsibilities needed to follow legal and ethical guidelines, such as GDPR or CCPA. This framework is also crucial to avoid security issues like data leaks and AI system bias, and to stay up to date with best practices in data governance, as the world of AI is still changing rapidly.
Step 4: Integrate AI in your systems
Finally, what we’ve been waiting for! After these preparation steps to help prevent error, you can move forward with selecting AI technologies to implement in your company. We recommend shopping around to find the best fit and looking for features that support data security, regulatory compliance, and scalability. Once you’ve made your decision, you can work to deploy the technology across your systems with your compliance framework.
Step 5: Train your teams on AI data governance principles
The importance of making sure your teams are properly trained on handling AI data can't be overstated. In these training sessions, you should emphasize data integrity and privacy and how your employees directly contribute to them. Creating individual responsibility in a larger team culture, whether it’s for engineers or administrators, can help prevent human error so that AI performs at its best. And because there are constantly new developments in how to govern AI, consider requiring regular training courses to help your teams brush up. Training programs and knowledge-sharing platforms should also be made available to foster a culture of data literacy and governance awareness across the organization.
Overcoming challenges in AI data governance
Of course, as with any new strategy, putting an AI data governance system into practice may come with a few hurdles. Here are a few common challenges and how to navigate them.
Data silos
It’s not uncommon that a company has existing data silos when deciding to implement an AI governance strategy. These silos must be broken down across departments in order to successfully integrate disparate data sources into a unified governance framework — but often, different departments have their own data management practices that make it difficult to create a cohesive strategy.
The best way to break down data silos for AI data governance is to foster collaboration between departments. This can help lead to standardization of data formats and consistency across data tagging and metadata practices. For additional measures, you can also consider implementing a unified data management platform.
Data privacy and security
Keeping data safe has never been an easy task, especially with the evolving methods that attackers use to access sensitive information. But data privacy is key for AI data governance, and companies should set up streamlined rules and guidelines for how sensitive information is collected, stored, and used. That means choosing bulletproof encryption techniques, access controls, monitoring tools, and auditing processes to ensure the integrity of your data.
Regulatory compliance
Equally as tedious as data privacy is navigating its complex web of regulatory requirements, such as GDPR and CCPA. Harnessing the full potential of AI while meeting these requirements is a delicate balance, especially when regulations vary across regions and are constantly changing. To combat this issue, you should consider appointing a dedicated data protection officer (DPO) to oversee compliance efforts and regularly update and educate your teams. Additionally, there are data management platforms that include regulatory compliance features, such as automated compliance reporting.
Scalability
Once you’ve implemented an AI data governance strategy, it must be scalable and flexible enough to accommodate future growth and changes in technology — without becoming overly restrictive, or worse, obsolete. Luckily, there are options to avoid this. You can leverage cloud-based solutions to help with scaling and allocate resources to continuously monitor and adjust your AI data governance strategy accordingly.
Cultural resistance
Lastly, your biggest challenge to implementing an AI data governance strategy may very well be your organizational culture. Employees can be reluctant to change their established processes or adopt new technologies, particularly if they don’t view AI as an asset to their workflows or see it as something that will stifle creativity. Overcoming this resistance requires strong leadership, clear communication, and a major emphasis on the benefits of AI data governance. You can accomplish this through employee training and by including key stakeholders early in the process to gain buy-in. You should also gradually phase in new processes and tools to allow for smoother adoption.
The future of AI data governance
AI data governance is positioned to revolutionize how organizations manage, secure, and deploy their data. And, the arena is changing fast thanks to new trends, enforcement mechanisms, and improving technology. It will increasingly remove manual work from data classification, compliance monitoring, and quality assurance while enhancing the ability to detect anomalies, enforce policies, and ensure that data is used ethically and responsibly across all areas of an organization. Moreover, AI will play a critical role in helping companies remain agile by offering predictive insights and real-time adjustments to governance strategies.
Emerging trends
Multimodal AI
Multimodal AI refers to artificial intelligence systems that can process and analyze multiple types of data inputs simultaneously, such as text, images, audio, and video. These systems can integrate information from various sources to gain a more comprehensive understanding and make more nuanced decisions. Multimodal AI is becoming increasingly important in areas like autonomous vehicles, virtual assistants, and advanced robotics, where the ability to interpret diverse data streams is crucial for effective performance.
Ethical AI
AI’s ability to govern data ethically by identifying and mitigating data bias has become a central focus for companies. We can expect to see AI data governance frameworks continue to develop guidelines and tools to ensure that AI-driven decisions are transparent and free of bias.
Explainable AI
Explainable AI (XAI) is a set of tools and frameworks designed to help users understand and interpret predictions made by your AI and machine learning models. This is becoming increasingly important as companies come to rely on AI — especially in areas like healthcare and banking that handle sensitive information. Explainable AI will help make data governance decisions clearer for all teams in an organization, and it will become more commonly used in compliance and risk management.
Real-time governance
There has been a notable rise in real-time data processing and analytics demand, and this is no different in AI data governance. AI tools are being developed to provide detailed data lineage and tracking instantly, allowing organizations to trace data throughout the data lifecycle, enforce data policies, and respond to issues as they arise.
Federated learning
Federated learning in the context of AI is a practice in which multiple entities train AI models while their data remains decentralized — differing from machine learning, in which data is centrally stored. This is generally motivated by data privacy concerns since it involves less data transfer and owners do not need to share raw data. But most of all, federated learning allows multiple parties to submit model edits without compromising data, meaning that teams can build smarter AI models together. This approach leverages AI’s strengths while ensuring human oversight and collaboration.
Conclusion
Integrating AI data governance into your organization can provide a huge strategic advantage in today’s digital landscape. By adopting a comprehensive strategy, you can improve the security and accuracy of your data while staying compliant with ever-changing regulations. Getting ahead of this opportunity will unlock new opportunities for growth and innovation and lead to smarter, more informed decisions and happier customers in the long run.
Fireside Chat: Adopt AI/LLMs Securely & Governably: Leverage Kong's expertise for safe innovation
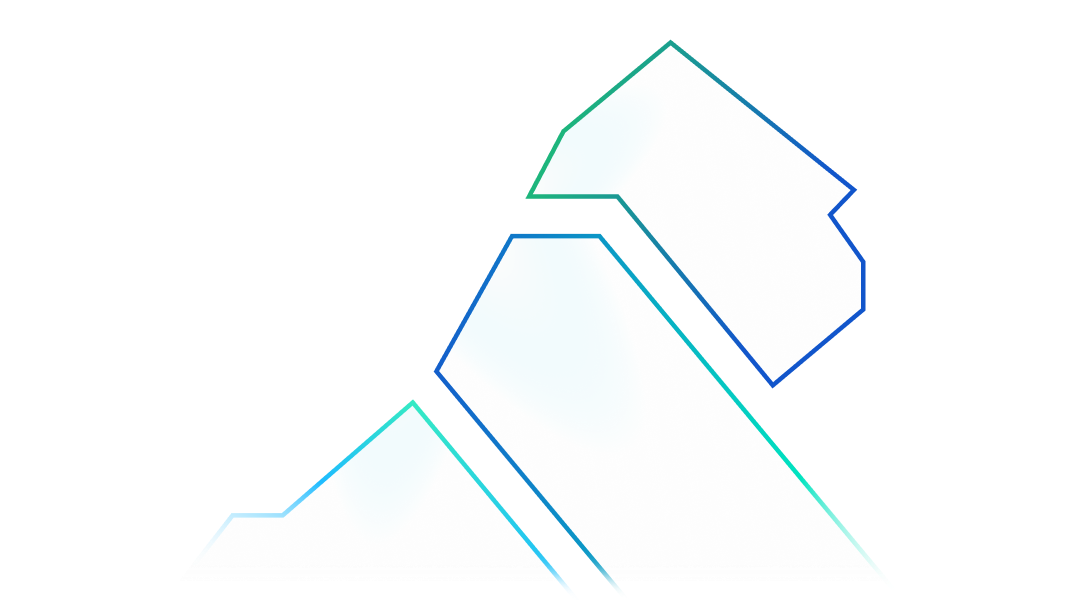