What is AI Governance? A Framework for AI Implementation
While AI is revolutionizing the future of nearly every industry, it’s also created a unique set of challenges and liabilities that will need to be addressed as the area grows. Enter AI governance: a set of rules and best practices to ensure that AI is used effectively, securely, and responsibly. But what exactly does that mean, and why is it so crucial for businesses?
When using AI, governance is essential to reduce bias, stay compliant and organized, and make decisions more transparent and replicable. Let’s break AI governance further and discuss its implications for modern organizations now and in the years to come.
The definition of AI governance
Think of AI governance as a rulebook for how to use AI in a secure, ethical, observable, moderated, and auditable fashion. It’s a systematic framework of policies and practices that governs the deployment, management, and optimization of AI data and technology within an organization.
Strong AI governance measures are designed to guarantee that AI systems are utilized legally and ethically, putting safeguards in place for the organization. This way companies can manage risks and be performant at the same time.
AI governance is broader than just ethical concerns. While ethical considerations are a core component, AI governance is essentially everything a company will need to consider if they want to implement AI throughout the company's different functions
AI governance use cases and examples
Not long ago, AI governance was just theory. Now, it’s rapidly shaping industries. Here’s how different sectors are tackling AI challenges.
AI in healthcare
AI has sent shockwaves through the healthcare industry, especially when it comes to handling patient care. However, due to the sensitive nature of patient data, which is a top priority for governing bodies, it requires strict oversight. Compliance measures like HIPAA are in place to ensure privacy and security for patients, and AI governance plays a big role in keeping data HIPAA-compliant. It also helps prevent bias in AI diagnostic tools so that all patients receive accurate and fair assessments. Finally, AI governance makes AI decisions more explainable, which is crucial because doctors and medical professionals need transparency to know they can trust AI-driven insights.
AI in finance
The financial sector relies on AI for critical functions like credit scoring, fraud detection, and risk management, but they must abide by fair lending practices as set by government regulations. AI governance requires AI models to be trained on diverse datasets to prevent discrimination and follow a clear line of decision-making to enable customers to understand why they are approved or denied credit. Furthermore, financial institutions must adhere to regulatory requirements, such as the Fair Credit Reporting Act, to maintain compliance and ethical standards.
AI in retail
AI has greatly enhanced personalized shopping experiences for customers and increased sales for many companies. AI governance helps to maintain consumer trust by ensuring data privacy policies so that customers know how their data is being collected and used by the retail company. AI also helps retailers avoid bias with AI recommendations, ensuring that product suggestions and promotions do not unfairly favor certain groups over others.
Fireside Chat: Adopt AI/LLMs Securely & Governably: Leverage Kong's expertise for safe innovation
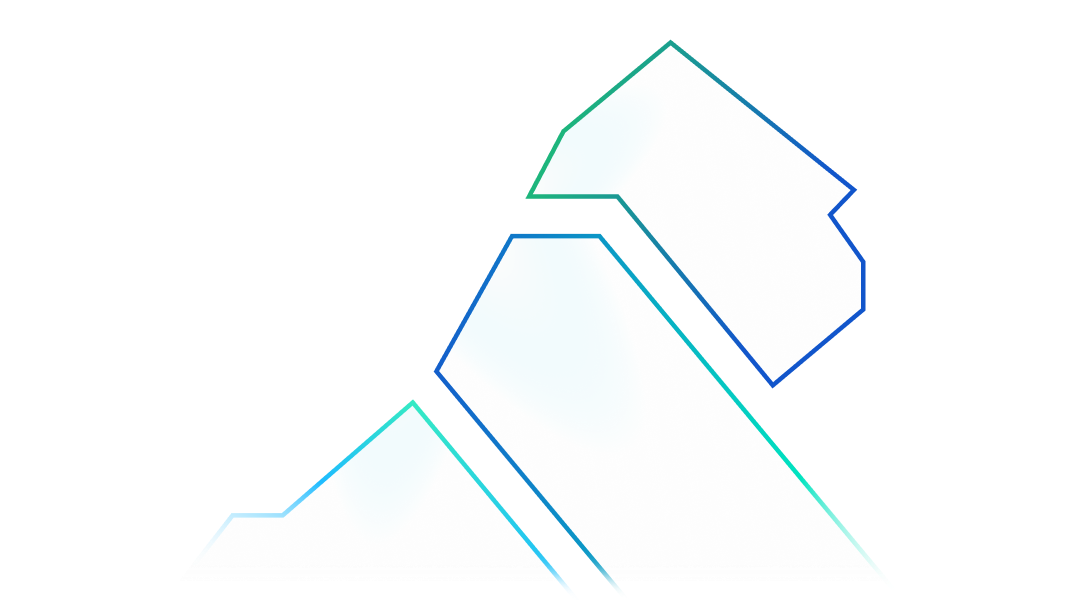
AI governance in development practices
AI governance can play a part in many common modern development practices.
- AIOps: AI-driven IT operations (AIOps) require strong governance to maintain maximum efficiency and compliance. Real-time monitoring can detect anomalies, trigger alerts, and automate remediation actions, while clear policies streamline incident response, root cause analysis, and continuous improvement.
- DevOps: Developers shouldn’t bypass integrating ethical AI practices into development operations (DevOps). Automated testing for bias, fairness, and transparency can be embedded into the continuous integration and delivery (CI/CD) pipeline using tools like IBM's AI Fairness 360 or Google's What-If Tool. These methods help developers enhance model transparency by generating human-readable explanations of model decisions and highlighting any areas of ambiguity.
Role of AI governance in all stages of the AI lifecycle
As AI becomes more prevalent across all industries, developers will need to understand how to deploy governance for ethical data use, risk mitigation, and transparency. Read on to learn how AI governance functions in different stages of the AI lifecycle for development.
- Data management: High-quality data is the bedrock of trustworthy AI. Governance practices such as data quality control, lineage tracking, secure data storage, and access management help ensure that data used for AI training and inference is accurate, complete, and consistent. Plus, encryption and role-based access control protect sensitive data from unauthorized access or breaches.
- Model development: In order to develop fair and unbiased AI models, there needs to be diverse training data, regular bias testing, and thorough documentation. Techniques like disparate impact analysis and sensitivity analysis help assess fairness by detecting bias and discrimination. The model development process should also include documentation of model architecture, training data, and evaluation metrics.
- Explainable AI: Transparency can't be overstated when it comes to establishing user trust and effective human oversight. AI models should provide clear explanations of decisions with feature importance scores or decision trees, and techniques like LIME (Local Interpretable Model-Agnostic Explanations) and SHAP (SHapley Additive exPlanations) can generate post-hoc explanations for black-box models. By making the AI model more intuitive and explainable, organizations can enable better decision-making and improve trust in AI outputs.
- AI security: Protecting AI systems from attacks is of utmost importance to maintain the integrity and confidentiality of AI data. Robust security measures like adversarial training defend against data poisoning and adversarial attacks from bad actors, as well as model stealing attacks, which attempt to extract the underlying model parameters or training data. Encryption, access controls, and regular security audits and penetration testing also help safeguard AI models by identifying and addressing any vulnerabilities in AI systems.
- AI testing and validation: No development work is complete without testing and validation. Every AI system needs regular, comprehensive performance testing to check its quality and reliability. Performance testing, bias audits, and user acceptance testing all validate AI outputs to protect user groups and ensure the AI meets real-world needs.
- AI lifecycle management: Governance must span the entire AI lifecycle, from design and development to deployment and retirement. This helps verify the system’s compliance with evolving regulations and standards. There should be clear policies and procedures for each stage of the AI lifecycle, including data collection, model validation, monitoring, and archiving. Organizations should also implement mechanisms for tracking and auditing compliance with specific relevant regulations and standards, such as GDPR or IEEE's Ethically Aligned Design. Taking a holistic approach to AI lifecycle management means organizations can ensure their AI remains safe, effective, and legally compliant.
Key pillars of AI governance
Ethical considerations
Ethical considerations make up the backbone of AI governance to ensure that systems are transparent, accountable, secure, and unbiased.
- Fairness and non-discrimination: AI should work for everyone, not just the select few who built it. Preventing bias means using diverse training data, consistently running bias tests, and fixing issues before they impact real users.
- Transparency: Transparent, explainable AI models allow users to better understand AI decisions and use the technology. Using decision trees or tools like LIME and SHAP can help make AI more transparent and easier to oversee.
- Accountability: Definitive governance structures assign individuals or teams to oversee system performance, enforce audits, and address threats or concerns. This ensures that parties are accountable to keep the AI functional and effective.
- Privacy and data protection: AI processes vast amounts of sensitive data. Strict governance protocols, like secure storage, data minimization, and privacy-first design, keep personal or private information safe and compliant.
Legal and regulatory frameworks
Another concern that goes hand-in-hand with ethics is compliance. Legal and regulatory frameworks like the GDPR (EU) and CCPA (US) set strict rules on the collection, management, and protection of personal and sensitive data. Organizations must justify data use, obtain clear consent, and enforce strong security measures.
Additionally, new laws are emerging to keep pace with AI advancements. The EU Artificial Intelligence Act and US Algorithmic Accountability Act push for greater transparency and accountability, placing restrictions on high-risk AI applications like facial recognition and automated decision-making.
Beyond national laws, global standards like the OECD AI Principles and IEEE Ethically Aligned Design provide ethical blueprints for AI governance. Aligning with these frameworks ensures compliance, builds trust, and promotes a unified global approach to responsible AI.
Risk management
There are certainly risks tied to the development and deployment of AI systems, and strong risk management protocols must be adopted in AI governance to identify, evaluate, and mitigate these risks.
- Identification: The first step in any risk management process is to identify potential dangers and bad actors, such as data privacy breaches, algorithmic bias, lack of transparency, or AI misuse. Risk assessments should consider the system’s unique context, application, and broad impact.
- Mitigation: Once risks to the AI system have been identified, organizations must take action to prevent or quickly respond to them. This means strengthening data protection, implementing techniques like access control, and setting clear policies for AI development and deployment. These strategies should evolve as new risks and environments emerge.
- Monitoring and review: Risk management doesn’t stop after deployment. AI systems need constant auditing, performance tracking, and stakeholder feedback to catch new risks early and get ahead of progress. Clear escalation and resolution protocols and transparent reporting are critical parts of the review process.
Stakeholder engagement
There have been concerns about how AI will impact individuals, businesses, and society at large. Because of this, governance must be a collective effort.
- Collaboration: Effective AI governance requires teamwork across governments, industries, academia, and civil society. Multi-stakeholder forums and public-private partnerships help create balanced, inclusive policies that address diverse concerns and opportunities in the AI sector.
- Public participation: AI governance often benefits from democratic processes, such as public input through consultations and feedback channels, either internally or externally. Such measures may help align AI systems with societal values, build trust, and uncover insights that even experts might miss.
- Stakeholder interests: As with any other endeavor, AI governance must also strike a balance with stakeholders. This includes combining innovation and safety, benefits and risks, and goals and needs. A well-balanced approach can drive progress while keeping ethics at the forefront of concerns.
How to adopt effective AI governance
Adopting effective AI governance is about creating frameworks that guide AI use in an ethical, transparent, and secure manner so that the technology can serve our best interests — not the other way around. The journey only just begins with setting clear policies and guidelines that govern how AI should be developed, deployed, and monitored so that everyone in the organization understands expectations and operates within ethical and legal boundaries.
Fostering a culture of responsibility is equally as important. Your teams should have a clear understanding of their specific roles and responsibilities. Additionally, investing in AI educational resources and training enhances AI literacy across the organization and empowers employees to make informed decisions and actively contribute to responsible AI initiatives.
By this point, it should be clear that transparency is the wheel that makes AI governance turn. Clear communication and processes build trust with consumers and stakeholders by helping them understand decision-making. This goes alongside embracing collaboration to bring the highest level of scrutiny and insights to AI governance. Organizations that provide cutting-edge knowledge and engagement opportunities see the greatest success in their AI governance strategies.
How Kong helps organizations adopt AI governance
Kong helps organizations achieve AI governance through its comprehensive suite of tools designed to manage, secure, and monitor API-driven applications and services, which support the architecture of AI systems. With these tools, Kong empowers organizations to navigate the complexities of AI governance in one place and scale up effortlessly.
- Secure and manage your APIs: Kong Gateway serves as a secure platform to connect to and manage LLMs, implementing authentication, authorization, and encryption protocols. Kong ensures that AI-driven APIs are accessed through a secure AI Gateway to comply with data protection regulations.
- Centralized control: With Kong Konnect, organizations can use a centralized platform to manage all their APIs and services, including those powered by AI. This unified control plane simplifies the oversight of complex AI ecosystems, ensuring consistent enforcement of governance policies across all AI services. By providing comprehensive visibility into the entire API landscape, Kong Konnect facilitates monitoring compliance with established AI governance frameworks.
- Modern observability: Kong provides extensive logging and monitoring capabilities to ensure that all interactions with AI services are transparent and traceable. This level of observability is essential for AI governance, as it allows organizations to audit AI behaviors, identify and mitigate biases, and implement accountability in AI decision-making processes.
- Scalability and performance: Built to scale, Kong's infrastructure supports the growth of AI services without compromising governance standards. This scalability means that as AI services expand, they remain compliant, performant, and reliable to meet the dynamic needs of businesses while adhering to governance requirements.
- Plugin ecosystem for custom AI governance: Kong’s AI Gateway leverages Kong’s extensive plugin ecosystem to meet a variety of AI governance needs. Organizations can implement a variety of pre-built plugins that enforce additional governance checks, such as AI model validation, bias detection, or ethical compliance checks, directly within the API gateway.
Conclusion
AI governance plays a pivotal role in shaping the responsible, ethical use of AI in today's ever-evolving digital landscape. By prioritizing key pillars like data privacy, transparency, and accountability, organizations can both mitigate risks and scale AI effectively and safely. A robust AI governance framework sets you apart as a reliable, forward-thinking leader, showcasing your commitment to customers, regulators, and stakeholders alike. This proactive approach helps build lasting trust and positions your organization for success in an AI-driven future.
Want to learn more about how to adopt AI and multiple LLMs in a secure, governable Way? Check out the AI Governance Playbook to see how innovation and security can be better balanced for responsible AI adoption.