Product Releases
June 26, 2025
Kong Konnect: Introducing HashiCorp Vault Support for LLMs
If you're a builder, you likely keep sending your LLM credentials on every request from your agents and applications.…

Marco Palladino
CTO and Co-Founder
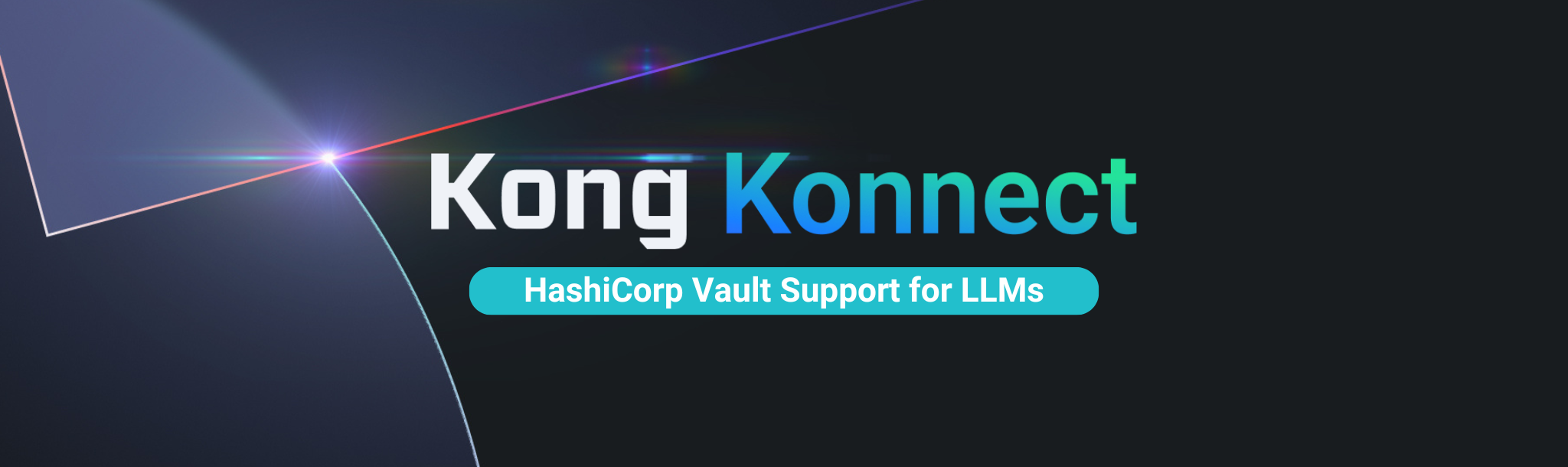
Talk tech with tips and tutorials from builders at Kong and the Kong Community.
Get insights from leaders changing business through API innovation.
Explore foundational guides unlocking key industry concepts and technologies.
Read up on what’s new and what's next in Kong’s latest product releases.